The Ultimate Machine Learning Software Review: Expert Insights and Recommendations
Introduction to Machine Learning Software
In recent years, machine learning has transformed from a niche field to a cornerstone of technological advancement. Whether you're a data scientist, a business leader, or an enthusiast, selecting the right machine learning software can significantly impact your projects and outcomes. In this review, we delve into the top machine learning software options, offering expert insights and recommendations to guide your decision-making process.

Key Features to Consider
When evaluating machine learning software, it's crucial to consider several key features that can enhance your workflow and results. Key aspects include:
- Ease of Use: User-friendly interfaces and comprehensive documentation can significantly reduce the learning curve.
- Scalability: The ability to handle large datasets and scale as your projects grow is essential.
- Integration: Seamless integration with other tools and platforms can streamline your processes.
- Support and Community: Access to customer support and an active user community can be invaluable for troubleshooting and knowledge sharing.
Top Machine Learning Software Options
The marketplace is brimming with machine learning software options, each with unique strengths and capabilities. Here, we highlight some of the top contenders:
- TensorFlow: Developed by Google, TensorFlow is renowned for its flexibility and comprehensive ecosystem. It's particularly favored for deep learning applications.
- PyTorch: Backed by Facebook, PyTorch offers dynamic computation graphs and is praised for its ease of use and robust performance in research and production.
- Scikit-learn: Ideal for beginners, Scikit-learn provides simple and efficient tools for data analysis and modeling without compromising on power.
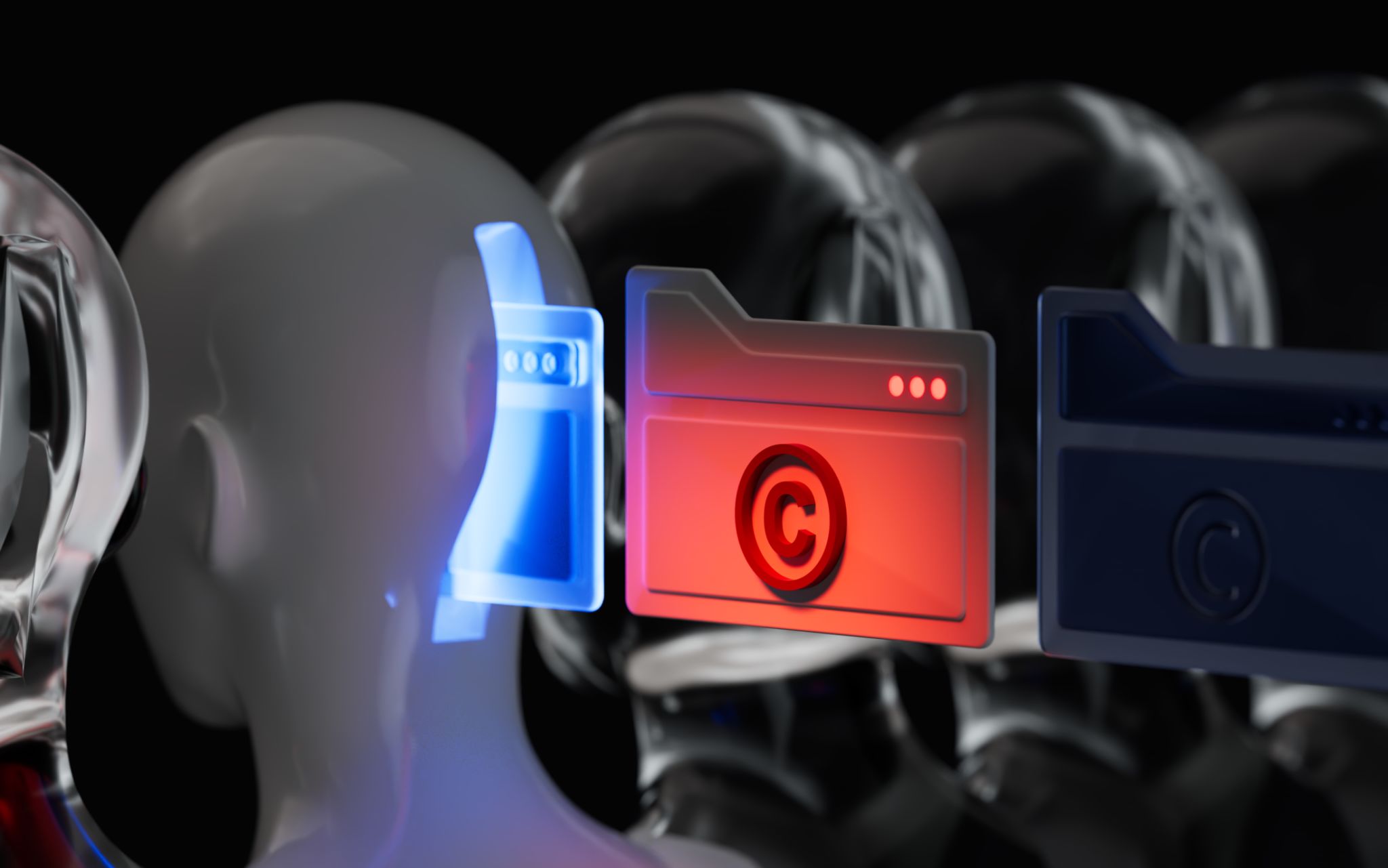
Expert Insights on Choosing the Right Software
Experts suggest that selecting the right machine learning software depends heavily on your specific needs and project goals. For organizations looking to build complex, scalable applications, TensorFlow's extensive features may be ideal. Meanwhile, if swift prototyping and iteration are priorities, PyTorch's intuitive design might be more suitable. For educational purposes or simpler tasks, Scikit-learn's user-friendly interface is recommended.
Real-World Applications
Machine learning applications span numerous industries, from healthcare to finance, each benefiting uniquely from different software capabilities. For instance, in predictive analytics within retail, Scikit-learn's straightforward approach can provide quick insights. In contrast, PyTorch's flexibility makes it a favorite in academic research for developing cutting-edge models.
Recommendations for Different Users
For beginners eager to explore machine learning, starting with Scikit-learn is often recommended due to its simplicity and abundant learning resources. Intermediate users might find PyTorch's balance of accessibility and functionality appealing. Advanced users with large-scale projects could leverage TensorFlow's powerful ecosystem to its fullest potential.

Conclusion and Final Thoughts
The world of machine learning is vast and rapidly evolving. Selecting the right software can set the foundation for innovation and success in your projects. By considering your specific needs and exploring the unique offerings of each tool, you can make informed decisions that align with your goals. Remember, the best software is one that not only meets your current requirements but also grows with you as your expertise and ambitions expand.
In conclusion, whether you're just starting or looking to elevate your machine learning endeavors, the right software is out there waiting to empower your journey into the future of technology.